UWSpace
UWSpace is the University of Waterloo’s institutional repository for the free, secure, and long-term home of research produced by faculty, students, and staff.
Depositing Theses/Dissertations or Research to UWSpace
Are you a Graduate Student depositing your thesis to UWSpace? See our Thesis Deposit Help and UWSpace Thesis FAQ pages to learn more.
Are you a Faculty or Staff member depositing research to UWSpace? See our Waterloo Research Deposit Help and Self-Archiving pages to learn more.
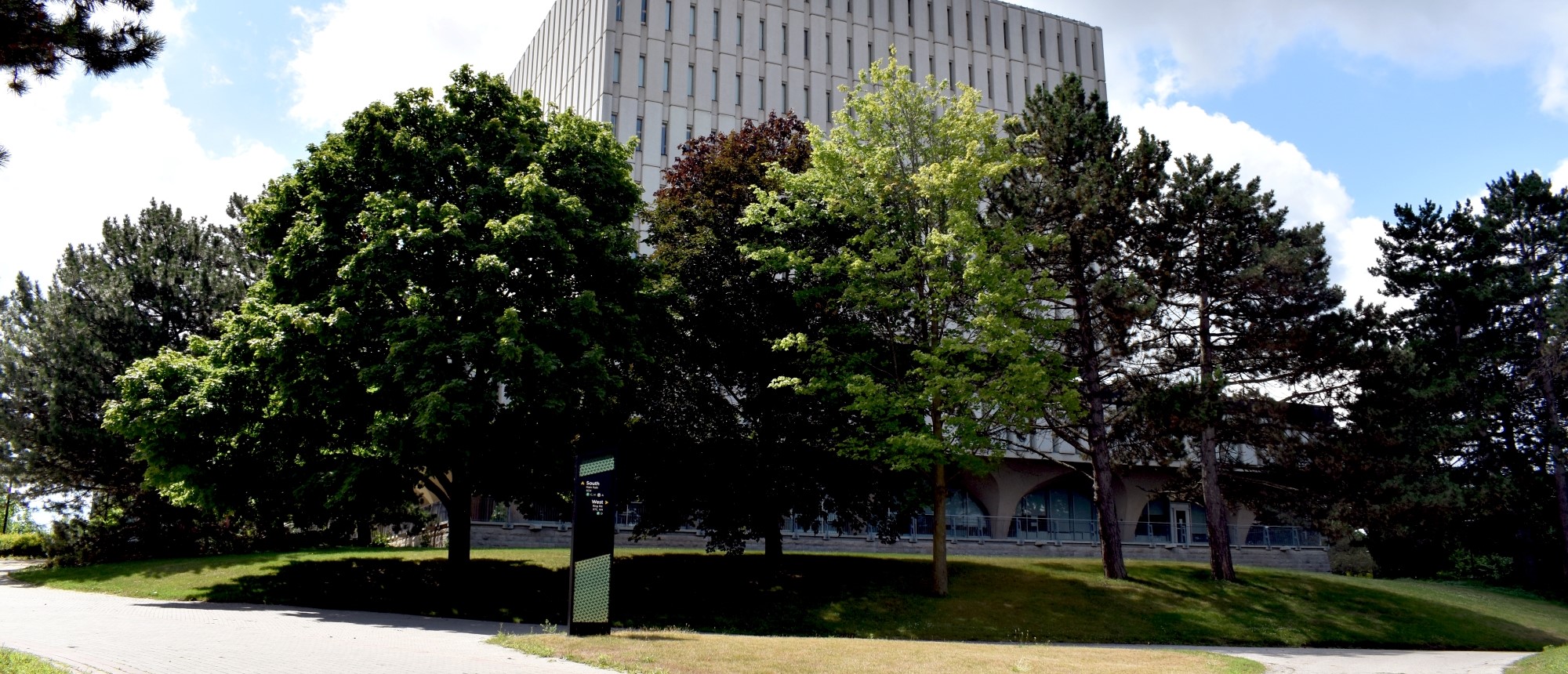
Communities in UWSpace
Select a community to browse its collections.
- The University of Waterloo institution-wide UWSpace community.
Recent Submissions
Neurovascular Coupling in healthy human retina evaluated with Optical Coherence Tomography
(University of Waterloo, 2024-12-21) Dhaliwal, Khushmeet
Retinal neurodegenerative diseases such as glaucoma, age-related macular degeneration (AMD), diabetic retinopathy, and retinitis pigmentosa affect millions of people worldwide and pose a significant burden on public health and the economy. Glaucoma, impacting approximately 80 million people globally, is a leading cause of irreversible blindness. In 2019, an estimated 19.8 million Americans (12.6%) were living with AMD, of which about 1.49 million people faced vision-threatening conditions. An estimated 9.6 million people were living with diabetic retinopathy in 2021, with about 1.84 million of them experiencing vision-threatening stages. In the United States alone, vision impairments- including those resulting from these retinal diseases- cost an estimated $139 billion annually.
Retinal neurodegenerative diseases not only cause progressive damage to the retinal morphology and vascular network, but also cause acute and transient metabolic, physiological, and blood flow changes at the early stages of the disease development which become permanent and chronic at the advanced stages of the disease. Neurovascular Coupling (NVC) refers to the transient vasodilation and increased retinal blood flow resulting from the increased metabolic activity of retinal neurons in response to visual stimulation.
Over the past few decades, a range of imaging techniques from clinical ophthalmoscope and confocal microscopy to adaptive optics scanning laser ophthalmoscope and optical coherence tomography (OCT) have been used ex vivo and in vivo to study components of the neurovascular coupling and its underlying mechanisms. Techniques such as Laser Doppler Velocimetry, Optical Coherence Tomography Angiography (OCTA), and Doppler Optical Coherence Tomography (D-OCT) have been used to observe the vascular responses of the retina caused by visual stimulation. Additionally, Electroretinography (ERG) has been widely used in clinical settings to evaluate the electrical activity of the neuronal retina. More recently, an optical equivalent to ERG, Optoretinography (ORG) was developed and OCT technology, imaging protocols, and image processing algorithms were designed to conduct OCT-based ORG studies in the human and animal retina. However, most of the Doppler OCT, OCTA, and ORG studies have examined components of the neurovascular coupling separately, potentially overlooking the dynamic interactions and comprehensive responses inherent in neurovascular coupling.
OCT, which acquires simultaneously both intensity and phase information, is particularly well-suited for investigating neurovascular coupling in the retina, as it enables a completely non-invasive approach for simultaneous monitoring of retinal blood flow dynamics and neuronal responses. The integration of a commercial ERG system with a research-grade OCT modality adds further value by offering easy control of the visual stimulus, use of clinically established ERG protocols designed to elicit responses from specific types of retinal neuronal cells, and using the ERG recordings to validate the visually-evoked neuronal responses. The main objectives of this PhD thesis were:
1. To develop a combined OCT+ERG imaging system to conduct in vivo and simultaneously morphological and functional imaging that can be utilized for investigating neurovascular coupling in the human retina.
2. To evaluate the performance and capabilities of the OCT+ERG system, imaging protocols, and image processing algorithms by conducting a pilot study on healthy human subjects.
3. To utilize the OCT+ERG technology to explore the neurovascular coupling mechanisms in the healthy human retina by extracting vascular and neuronal responses from different retinal layers simultaneously.
4. To examine the effects of different wavelengths and flicker frequencies on the dynamic retinal blood flow changes evoked by visual stimulation, providing deeper insights into the mechanisms of neurovascular coupling.
Results from this PhD research have been summarized in three manuscripts that are either under review or under preparation for submission. Therefore, this PhD thesis was prepared in such a way that individual manuscripts represent separate thesis chapters.
From Fisher Economicus to Fisher Socialis: Investigating the Role of Fisher Behaviour for Effective and Equitable Governance
(University of Waterloo, 2024-12-20) Battaglia, Maria Bernadette
The purpose of this dissertation is to advance the emerging field of fisher behaviour. The applied aim is to enhance fisheries and oceans policy, and primarily within the context of small-scale fisheries sustainability. In this dissertation, fisher behaviour is defined as the actions (or inactions) taken by fishers in response to internal and external stimuli and it describes the multitude of ways in which fishers interact with their social and ecological environment. Further, fisher behaviour can manifest through physical action, verbal expression, emotional responses, and cognitive processes.
Fisheries and oceans policy shapes and is simultaneously shaped by fisher behaviour because regulations and enforcement mechanisms that emerge from policy interventions signal what behaviours are allowed and which ones are not. Fishers, in turn, interpret these signals based on their values, needs, and perceptions of legitimacy over resource use. For this reason, when policy does not adequately reflect the diverse range of factors that shape fisher behaviour, regulations can become inefficient or inequitable and may result in poor social and ecological outcomes.
Despite the importance of behaviour and its central role in the pursuit of fisheries and oceans sustainability, fisher behaviour is complex and hence still not well understood. Historically, predominant paradigms of behaviour have been based in neoclassical economics’ Homo Economicus. These models of behaviour predict that rational and self-interested individuals will always prioritize personal gain over the collective interest and, without external interventions, they will inevitably deplete shared resources. Yet, in the last few decades, empirical evidence has challenged these assumptions, and has shown that resource users, including fishers, are able and willing to engage in collective action to solve social dilemmas.
The scope of this dissertation is to use the emerging field of fisher behaviour as a critical lens to strengthen fisheries and oceans policy. To achieve this overarching aim, this dissertation is guided by three interrelated research objectives: 1) To advance and understand fisher behaviour as an emergent and critical, yet understudied, field through the development of a comprehensive conceptual typology based on selected literatures; 2) To map and synthesize the complex interactions between social norms (as a particular manifestation of fisher behaviour), collective action problems, and fisheries policy and to further unpack the role of social norms as a catalyst of collective action in natural resource systems, including in fisheries systems; and 3) To empirically examine the role of social norms and social networks as two fisher behavioural approaches and further assess their implications for policy.
The first objective provides the foundation of this dissertation and frames the context and significance of this research by presenting an overview of alternative behavioural approaches to understand fisher behaviour. The second and third objectives delve deeper into two behavioural approaches, and in doing so, they challenge one of the core assumptions of Homo Economicus: that humans are self-interested, hence incapable of solving collective action problems.
The research methodology used in this dissertation is informed by deductive and inductive approaches and the integration of qualitative and quantitative methods, alongside a theoretical exploration. To further understand fisher behaviour in real world settings, a case study was conducted in Sardinia, Italy, in the context of three small-scale fishing communities adjacent to the Asinara MPA. Fieldwork was conducted both in-person and coordinated remotely in response to COVID-19 pandemic requirements. Research instruments were co-developed in collaboration with BMF, a not-for-profit organization working in the area, and further reviewed by key community informants to ensure coherence with the local context. Further, informal conversations with research partners and members of the local communities added additional depth and confirmed the direction of the research findings.
In Chapter 2, a theoretical exploration of existing literature on fisher behaviour was adopted to provide a typology of key selected approaches that offer an interdisciplinary suite of entry points and complementary opportunities to advance the understanding of fisher behaviour. These approaches include theories, concepts, and perspectives from Critical Social Theory, Systems Approaches, Development Scholarship, and Institutional Scholarship. Two vignettes, one based in Italy, and one based in Canada, were included in this chapter to add further empirical weight to the chapter by delving deeper into two of the lenses presented in the typology.
In Chapter 3, a systematic scoping review was used to map and synthesize existing literature on social norms in fisheries. However, given the limited availability on empirical articles that focused solely on fisheries, the scope of the evidence synthesis was broadened to include other environmental contexts. As such, the systematic scoping review was conducted on peer-reviewed articles (n=69) to map and synthesize ways in which social norms are conceptualized, elicited, and measured in the empirical literature at the intersection of social norms and collective action problems in environmental settings. This evidence synthesis followed the PRISMA-ScR. Findings revealed that social norms can be conceptualized as collective or individual constructs, and they can be elicited or measured using a variety of qualitative and quantitative methods. Further, social norm definitions do not necessarily correspond to a unique elicitation method. These results suggest that what may initially appear as lack of coherence is instead an opportunity to study social norms from various angles and perspectives.
In Chapter 4, structured surveys (n=81) were used to empirically examine the role of social norms and social networks in the Asinara MPA communities. Findings indicated that the existence of subgroups within networks does not necessarily hinder capacity for collective action, as analyses on the strength and distribution of social norms showed that cooperative behaviours within the Asinara MPA communities were still strong. Importantly, network analyses also elicited the presence of well-connected, central actors within each subgroup. This finding holds promising potential for collective action because central figures can leverage their positions to synthesize subgroup heterogeneity and generate innovative solutions to shared challenges.
Findings and insights of this dissertation contribute to advancing the emerging field of fisher behaviour, while offering alternative approaches to the paradigm of behaviour based in Homo Economicus. Context-relevant knowledge on fisher behaviour can be operationalized in policy settings to catalyze change using insights on who fishers are and the reasons behind their actions. For instance, Challenge 10 of the United Nations 2021-2030 Decade of Ocean Science for Sustainable Development aims to identify barriers to behaviour change to achieve ocean health. However, this aim cannot be achieved using models of human behaviour that underserve and oversimplify the complexity of empirical reality.
Findings from this dissertation translate into theoretical and empirical contributions by helping identify lenses and approaches to enhance fisheries and oceans policy, through a more comprehensive understanding of fisher behaviour. These insights can support policy in three complementary ways. First, knowledge on fisher behaviour can enhance coherence between policy and the social context within which policy instruments are embedded, which includes the behavioural elements of social systems, such as fishers’ values, needs, and beliefs. Second, aligning policy with contextual knowledge about fisher behaviour can improve policy equity by bringing recognition to pre-existing forms of organization (e.g., social networks) and informal rules of behaviour (e.g., social norms) that fishers have developed over long periods of time and persistence. Finally, this research reveals that there are currently untapped opportunities to generate new evidence about fisher behaviour. However, these efforts will require challenging the assumptions that have long underpinned fisheries and oceans policy, and cultivating collaborations across academia, policy practitioners, and fishing communities to inform the development of new methodologies and contextually-relevant understandings.
Computational study of cellular adhesion in metastasis: Implications for Circulating Tumor Cell Arrest, Extravasation, and Thrombosis Formation
(University of Waterloo, 2024-12-20) Rahmati, Nahid
Cancer metastasis is the process by which cancer cells spread from the primary tumor to distant sites in the body, forming secondary tumors. This process is responsible for the majority of cancer-related deaths, despite significant advancements in treating primary tumors. This thesis aims to enhance the understanding of metastasis mechanisms by exploring the roles of circulating tumor cells (CTCs), ultra-large Von Willebrand Factor (UL-VWF) multimers, and blood vessel configurations. This study focuses on the mechanical, biochemical, and hemodynamic factors that drive metastatic processes and cancer-associated coagulopathies, providing insights into the interactions between CTCs, VWF, and endothelial cells.
Through computational modeling and simulations, first, we investigate the role of UL-VWF multimers in cancer-associated thrombosis. The computational model integrates the lattice Boltzmann method for simulating blood flow, a coarse-grained model for deformable cells to capture their mechanical behavior, and the immersed boundary method to handle fluid-structure interactions. Additionally, an adhesion model was developed to simulate the binding dynamics between cells. This multi-scale approach allows for a detailed analysis of how UL-VWF multimers interact with blood cells to initiate microthrombus formation and progression. The findings reveal that UL-VWF plays a dual role in thrombosis and metastasis, enhancing platelet adhesion and trapping red blood cells, which can lead to significant changes in blood flow dynamics, such as reduced velocity and increased shear stress near thrombus sites, leading to a pressure drop of up to six times compared to healthy conditions.
The study also explores the impact of blood vessel architecture on CTC dynamics, focusing on how vessel tortuosity influences CTC adhesion and extravasation. The same computational methodology has been utilized to analyze CTC interactions with the vessel wall, incorporating adhesion dynamics between the CTCs and the endothelial surface while considering the effect of shear rate on adhesion strength. The results indicate that curved vessels create asymmetrical flow patterns, resulting in variable shear stress, a 25% decrease in the wall shear stress in low-shear regions and a 58.5% increase in the high-shear region, that significantly affects CTC behavior. Specifically, high-shear regions in curved vessels show a threefold rise in adhesion bond formation compared to straight vessels, enhancing the likelihood of CTC extravasation. Increasing the tortuosity index of the vessel led to a 50% increase in maximum wall shear stress ratio and a 15.3% decrease in minimum wall shear stress ratio, as well as a 58% increase in the transit time of CTCs through the vessel curvature. The adhesion force in these high-shear regions increased by about 171%, indicating a significantly higher risk of CTC adhesion and extravasation in vessels with higher curvature. Additionally, while softer CTCs in low-shear regions showed a higher likelihood of detachment, stiffer cells in high-shear regions exhibited a reduction of approximately 12% in adhesion force compared to their behavior in straight vessels. This study identified an optimal range of cellular stiffness for successful CTC extravasation, challenging the assumption that softer cells always extravasate more efficiently.
In this thesis, we also employed a stochastic model to analyze the dynamics of CTC adhesion, a crucial factor driving metastasis, incorporating parameter uncertainties in cell mechanical properties and adhesion characteristics. This probabilistic approach realistically captures the biological variability inherent in CTC behavior by accounting for a wide range of possible cell adhesion scenarios. Our analysis revealed that incorporating parameter variability, with a coefficient of variation of 20%, led to a maximum uncertainty of 12% in cell velocity. This variability manifested in two distinct CTC behaviors: either the cells detached from the vessel wall or continued to roll in a semi-stable manner, emphasizing the non-linear and complex nature of the adhesion dynamics. To efficiently manage computational demands, we developed a Random Forest surrogate model, achieving a high level of accuracy with a maximum error of 4.36% for velocity and 0.63% for stretch ratio. This model enabled comprehensive sensitivity analysis using Sobol' and E-FAST methods, which identified the bond spring constant and rupture strength as the most influential parameters following the initial adhesion phase, while cell membrane elasticity played a critical role during the initial adhesion. We also observed significant interdependencies between bond formation and rupture properties, underscoring their combined impact on CTC dynamics. Furthermore, machine learning techniques, particularly XGBoost, validated the model's predictive capabilities by achieving a classification accuracy of 95.62% and an area under the curve (AUC) value of 0.99 in distinguishing between 'rolling' and 'detached' CTC states. These findings highlight the importance of focusing on key parameter interactions to refine predictive models for metastasis.
This comprehensive approach builds on the computational frameworks developed in this thesis, enhancing our understanding of metastasis by offering predictive insights into CTC behavior under different conditions. By integrating these findings into a cohesive framework, the thesis supports the development of more targeted therapeutic strategies to prevent or disrupt cancer progression.
A Community-Based Exploration on the Impacts of Land-Based Learning in an Urban Indigenous Community
(University of Waterloo, 2024-12-20) Bui, Danica
Background
Connection to the Land is essential for Indigenous Peoples’ health and well-being. Indigenous Food Sovereignty works towards regaining control over traditional food systems. There is a need for sustainable, Indigenous-specific, and accessible urban community Land-based practices. Done in partnership with White Owl Native Ancestry Association (WONAA) and the Wisahkotewinowak Collective (WC), four seasonal Land-based learning events were held. Each event involved a workshop, meal, and gathering at the University of Waterloo’s North Campus Community Gardens.
Aims
Guided by the interests of project partners, WONAA and WC, the overall purpose of this thesis is to explore and evaluate the impacts of Land-based learning on the local Indigenous and university communities and the PEG project’s effectiveness in establishing networks to facilitate ongoing research and learning to establish a permanent Land-based learning space on campus.
The overarching research questions that guided the research are:
1. What are participants’ and facilitators’ perceptions of the Land-based engagement process?
2. What is the effectiveness of Land-based workshops in identifying and prioritizing collaborative Land-based opportunities for learning and research?
3. To what extent can the seasonal workshops establish a local network to facilitate ongoing research and learning in support of the development of an urban Indigenous Land-based learning space on the UW campus?
Methods
Appreciative Inquiry and Community-Based Participatory Research approaches were used for this thesis. Following each of the events, participants and project collaborators were recruited to participate in interviews. The data for this evaluation was collected through 17 semi-structured interviews with project collaborators and community partners (n=6), urban Indigenous community members (n=6), Indigenous youth and students (n=2), and UW staff (n=3), two focus groups with WONAA and WC staff members (n=5) that were involved with the workshops, and a document review. Reflexive thematic analysis was completed using NVivo for the interview and focus group transcripts, and the documents were analyzed to determine if the budget and timelines were met.
Results
Overall, participants, collaborators, and facilitators of the Land-based learning events had positive perspectives of establishing a Land-based learning space on campus. The three key themes from the interviews about the Land-based learning events and their potential for building connections to establish a permanent Land-based learning space include: (1) participants’ growing support of Land-based learning (2) creating connections and building relationships and (3) institutional support and project sustainability to support of the development of a permanent urban Indigenous Land-based learning space. The findings from the outcome evaluation, including the interviews, focus group, and documents reflect these themes and include (1) workshop feedback (2) project partners' goals, and (3) identifying opportunities to move forward. These findings show that the community, including project partners, participants, and collaborators, found the Land-based learning events to be a welcoming and positive experience that should become integrated into university curricula to support the university and local community.
Conclusion
Participants enjoyed the Land-based learning events and supported the establishment of a permanent Land-based learning space at the North Campus Community Gardens. Attending the events and being involved in the project generated ideas for learning and research opportunities, with many participants sharing future workshop ideas and identifying ways to integrate Land-based curricula and research opportunities. The events also connected the local urban- Indigenous community to the university community and helped to strengthen pre-existing relationships between the attendees. Through these connections, the desire for the North Campus Community Garden to become a space for Indigenous learning, teaching, and research, strengthened. Overall, the interview and focus group participants had positive perspectives of the events and the establishment of a permanent Land-based learning space at the North Campus Community Gardens, shared that Land-based learning was a need in the urban Indigenous and university community, and emphasized that further support from UW, longer-term funding, and physical and social infrastructure was needed for the project’s sustainability.
A Two-Effects Model of Explanation on Exposing the Illusion of Understanding
(University of Waterloo, 2024-12-20) Meyers, Ethan
People often overestimate their understanding of how things work. For instance, people believe that they can explain even ordinary phenomena such as the operation of zippers and speedometers in greater depth than they really can. This is called the illusion of understanding (originally known as the illusion of explanatory depth). Fortunately, a person can expose the illusion by attempting to generate a causal explanation for how the phenomenon operates (e.g., how a zipper works). This might be because explanation makes salient the gaps in a person’s knowledge of that phenomenon. However, recent evidence suggests that people might be able to expose the illusion by instead explaining a different phenomenon. Across six preregistered experiments and one secondary data analysis, I examined whether explaining one phenomenon (e.g., how a zipper works) leads individuals to lower their self-assessed knowledge of unrelated phenomena (e.g., how snow forms). My findings demonstrated that participants consistently revised their understanding downwards, not only for the item they explained but also for other items they did not explain. For instance, participants reported reduced understanding of speedometers after explaining helicopters or zippers. Contrary to prior research, participants did exhibit the illusion for familiar movie plots (Experiment 4), but consistent with prior research, participants did not exhibit the illusion for common procedures (Experiment 5). Additionally, when common procedures were included in the experimental design used in Experiments 2 and 3, participants showed no illusion whatsoever (Experiment 6). Finally, an analysis of explanation quality using ChatGPT to code the explanations revealed that the reduction in perceived understanding after explaining (compared to before) correlated with the difference between how well the participant thought they understood the item and how well they actually explained it, but only for explained items. These findings challenge the common framework of how the illusion of understanding operates. Throughout the thesis I evaluate alternative models of the illusion and ultimately find the most support for a two-effects model of explanation, wherein failing to explain a phenomenon temporarily makes people recognize the gaps in their knowledge of the item they explained and makes them feel less knowledgeable about most other things.