UWSpace
UWSpace is the University of Waterloo’s institutional repository for the free, secure, and long-term home of research produced by faculty, students, and staff.
Depositing Theses/Dissertations or Research to UWSpace
Are you a Graduate Student depositing your thesis to UWSpace? See our Thesis Deposit Help and UWSpace Thesis FAQ pages to learn more.
Are you a Faculty or Staff member depositing research to UWSpace? See our Waterloo Research Deposit Help and Self-Archiving pages to learn more.
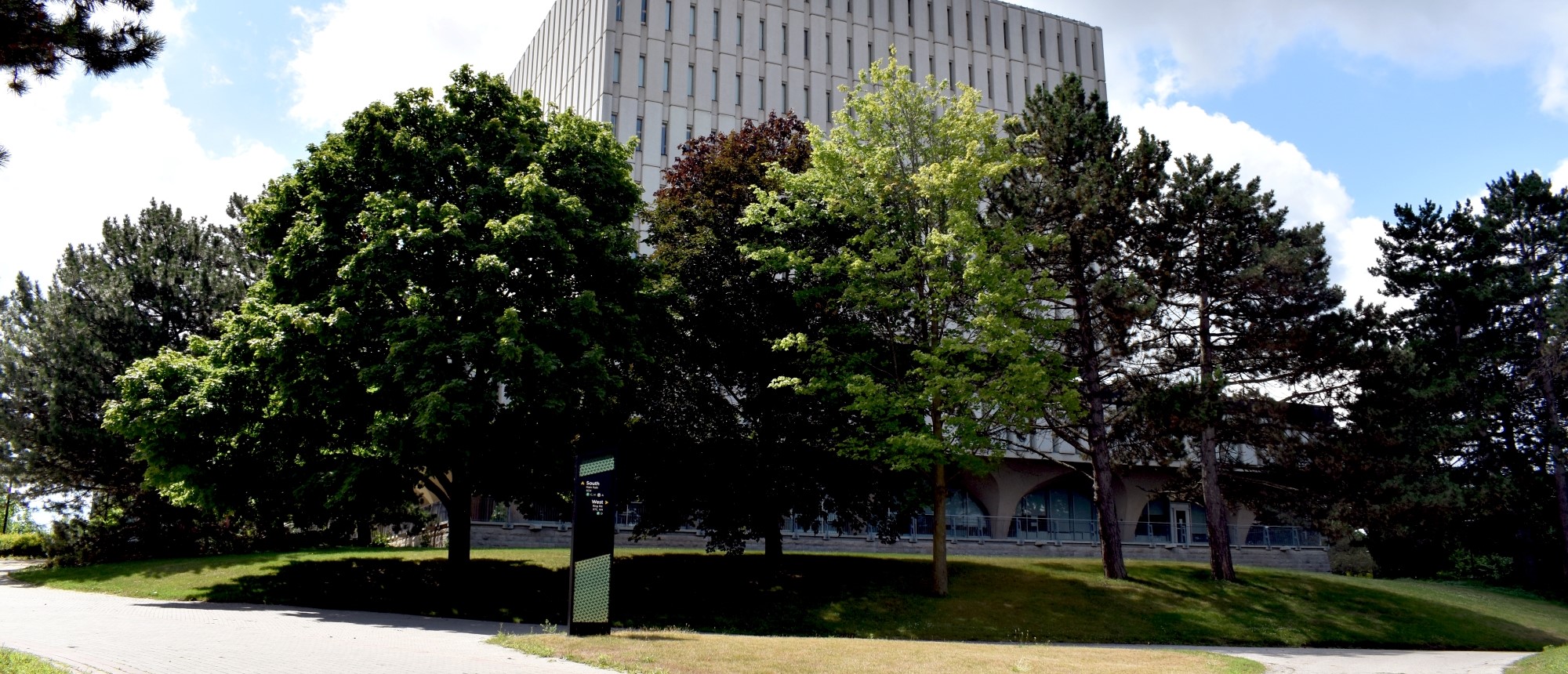
Communities in UWSpace
Select a community to browse its collections.
- The University of Waterloo institution-wide UWSpace community.
Recent Submissions
On the three charge regimes of bipolar charge conditioners
(Taylor & Francis, 2025-03-12)
Many aerosol instruments utilize bipolar charge conditioners (neutralizers), including scanning mobility particle sizers (SMPS). The charge distribution from a bipolar charge conditioner must be known to calculate size distributions from SMPS scans. Therefore, a reproducible and known “steady-state” charge distribution is desired to improve measurement accuracy. In this work, we show that although a steady-state charge develops within a common Kr-85 bipolar charge conditioner (TSI 3077A), gaseous ions are readily convected into tubing downstream of the charge conditioner, causing significant deviation from steady-state in less than a second. The “downstream ions” are predominantly positive since negative ions are more readily lost to tubing walls due to their higher diffusivity. Others have previously studied this potential effect, but there is disagreement among them. This study resolves the disagreements, and to the authors’ knowledge, we are the first to: quantify the surprising significance of this effect (mean charge at 239 nm changes by up to a factor of 4); show it occurs rapidly (milliseconds); and demonstrate that a true steady-state distribution is more asymmetric than classical theory (mean charge at 239 nm is >3x higher in magnitude). Interaction time of the particles with the remaining free ions downstream of the charge conditioner is shown to be the main consideration for this effect, as demonstrated by varying flow rate, tube length and tube diameter and achieving similar charging results as a function of this time. We perform advanced numerical modeling of charging, convection and diffusion of particles and ions, and show good quantitative agreement with experimental data. These results are further supported by similar charging results measured from a bipolar charge conditioner that has a different internal geometry and ion source than the 3077A charger. To increase consideration of this critical charging effect and enhance understanding of bipolar charging in general, we thereby quantify three distinct regimes of bipolar charging, namely: the (1) charging regime (i.e., as charge develops from its initial to steady-state), (2) steady-state regime, and (3) discharging regime (i.e., effect of “downstream ions”). These three regimes of bipolar charging have wide implications for designing instrumentation, interpreting measurements and validating charging models with the aim of improving accuracy in aerosol instruments such as the SMPS
The Voice that Travels from Vessels to Other Worlds: Extracting Narratives from the Cave of the Sibyl
(University of Waterloo, 2025-04-02)
From caves, leaves, dust, to ampullae, the Sibyl of Cumae’s voice has travelled far from vessels and into other story-worlds in various material forms. While practicing as a female prophet in classical antiquity, her origin possesses no definite beginning but only allusions to an incomplete identity and liminal persona. The Sibyl of Cumae’s uncertain characteristics have rendered her an ideal figure for speculative interpretation over time, particularly through stories. Based on Vergil’s famous account in the Aeneid, the Sibyl of Cumae was imagined to be dispersing prophecies from inside a subterranean architecture: a cave in the ancient city of Cumae, Italy. With increasing interpretations of the Sibyl dealt through time, writers from the past have provided her material presence while oncoming generations continue to mold it to create something new amongst her absence. This thesis will critically examine the evolution of the Sibyl of Cumae’s character in a selection of seven texts from two separate timelines. Reading Vergil’s Aeneid, Ovid’s Metamorphoses, Petronius’s Satyricon, Mary Shelley’s The Last Man, Gerard Manley Hopkins’s Spelt from Sibyl’s Leaves, T.S. Eliot’s The Wasteland, and Sylvia Plath’s The Bell Jar, I find varied interpretations of this Sibyl united by a shared theme of isolation. Isolation, or the condition of separateness, becomes a motif to study in this thesis through form, material, emotion, and narrative. I unravel varied meanings and representations of isolation in the selected texts by examining the qualities of objects and architecture that have been associated with the Sibyl of Cumae in them including the cave, dust, ampulla, leaves, cage, and jar. I distinguish the qualities of decay, silence, and visibility from these objects and study their prominence across settings, characters and objects in the selected texts. This leads me to recognize how the Sibyl of Cumae has adapted into something beyond a corporeal presence by proxy of material entities of the physical world.
Can Social Robots Improve People’s Attitudes towards Individuals who Stutter?
(ACM, 2025)
Public attitudes towards stuttering are rooted in stereotypes and misconceptions, leading to negative reactions and discrimination against individuals who stutter. Previous research highlights the positive impact of educational interventions on people’s attitudes towards stuttering. The potential of social robots as an educational tool in the context of stuttering awareness remains unexplored. In the present study, we investigate whether a social robot can improve public attitudes when giving an interactive presentation on the topic. We compare its impact with a tablet-only condition. Additionally, we differentiate between two robot conditions - one in which the robot imitates stuttering and another where the robot has fluent speech. In the robot conditions, visuals are shown on a tablet.We used a co-design approach and incorporated the perspectives and experiences of two individuals with lived experiences of stuttering into our study design. A user study with 69 participants reveals significant improvements in attitudes across all three conditions, with no significant difference between conditions. However, participants perceived the robot as significantly ‘warmer’, more ‘attractive’ and ‘novel’ when compared to the tablet. These findings provide valuable insights into the potential of social robots as intervention techniques for improving attitudes in the field of stuttering.
Mitigating Privacy Harms from Deceptive Design in Virtual Reality
(University of Waterloo, 2025-03-15)
This report was prepared as a final project report in response to the Office of the Privacy Commissioner of Canada Contributions Program 2024-25.
This research investigates deceptive design in Virtual Reality (VR) environments and its impact on user privacy. The research team at the University of Waterloo conducted an autoethnographic evaluation of 12 top-rated VR applications to analyze deceptive design patterns in their privacy communication and interaction mechanisms. The findings reveal 14 distinct deceptive design patterns and demonstrate how deceptive tactics in traditional web and mobile environments are being adapted to immersive environments. While many of these patterns still heavily rely on 2D interfaces elements, VR’s spatial, immersive, and multi-sensory features amplify their impact and increased the privacy risks for users. The VR applications’ convoluted privacy policies and consent mechanisms further hinder user comprehension on the data practices. Through the evaluation, the team also identified 7 exemplary privacy-enhancing design strategies, which can serve as a foundation for improved implementation of privacy mechanisms in VR environments.
To assess user perceptions of these deceptive design patterns, the team surveyed 424 users of the selected VR applications. The findings indicate that while users felt the manipulative influences and expressed discomfort, they often resign themselves to accepting privacyinvasive options and viewing such design as “typical” or “inevitable” across both VR and nonVR platforms. Their repetitive exposure to such design in mobile and web environments fostered a false sense of normalcy, and eroded user resistance to manipulation.
This research provides valuable insights for VR developers, designers, policymakers, and researchers on creating privacy-preserving VR experiences and developing clearer, more ethical privacy policies in this rapidly evolving field.
Coronavirus, Climate and a Clean Energy Transition: Is Resiliency Achievable?
(Balsillie School of International Affairs, 2020-04-02)
In the seeds of this current tragedy lies a historic opportunity for Canada to transition to a low-carbon energy economy — away from dependence on the oil and gas sector.